- The Aurorean
- Posts
- #34 | Projections To Clean The Ocean
#34 | Projections To Clean The Ocean
+ an eyeball transplant, a paradigm shift in AI reasoning and more
Hello fellow curious minds!
Welcome back to another edition of The Aurorean.
There are so many great stories for us to cover, we’re going to get right to it and not waste any of your precious time and our limited newsletter real estate.
Wondering what STEM discovered last week?
Let’s find out.
Quote of the Week 💬
Projected Plan To Eliminate The Great Pacific Garbage Patch
“Today’s announcement is clear: clean oceans can be achieved in a manageable time and for a clear cost… We call upon the world to relegate the Great Pacific Garbage Patch to the history books. This environmental catastrophe has been allowed to exist, unresolved, for too long, and for the first time, we can tell the world what it costs, what is needed and how long it could take. It is time for action.”
⌛ The Seven Second Summary: The Ocean Cleanup organization recently announced a plan to remove floating plastic pollution from the Great Pacific Garbage Patch within 10 years at a cost of $7.5 billion.
🔬 How It Was Done:
The team is using a 1.6-mile-wide U-shaped floating barrier system that is towed by two vessels at walking speed throughout the ocean.
The system collects floating debris into a central retention zone, which is then pulled onto the ship's deck for sorting and recycling.
Multiple wildlife safety measures are in place to minimize the damage to aquatic life, such as the slow vessel speed to help fish avoid the machine, underwater cameras and lighting to monitor wildlife encounters, and a remote safety hatch to help wildlife escape the vessels if they get caught.
🧮 Key Results: The team estimated a baseline, conservative and aggressive target for this cleanup operation based on their average historical performances at cleaning up smaller patches of water around the world. If they are able to operate with enough speed and efficacy, The Ocean Cleanup projects it is possible to complete the cleanup in 5 years at just $4 billion.
💡 Why This May Matter: Estimates suggest removing The Great Pacific Garbage Patch can expel 90 kilotons of CO2 into the atmosphere annually, which is equivalent to the emissions from a town of 3,300 residents. This is possible because the ocean, algae and phytoplankton all absorb CO2 from the atmosphere, and removing debris from the surface helps the planet regulate its climate more effectively. Less debris also protects wildlife from danger and the global food supply from contamination.
📚 Learn More: The Ocean Cleanup. YouTube.
Stat of the Week 📊
An Update On The World’s First Transplant Of An Entire Eyeball
1st
⌛ The Seven Second Summary: Surgeons at NYU Langone Health performed the world's first eyeball and face transplant on a 46-year-old man who suffered catastrophic injuries from a high-voltage electrical accident in 2023.
🔬 How It Was Done:
The medical team transplanted an entire left eyeball, as well as the eye socket, nose, chin bone, optic nerve, muscles, and blood vessels from a brain-dead donor to the recipient over the course of a 21-hour surgery.
The surgeons used 3D-printed surgical guides to help them take precise amounts of bone and tissue from the donor to fit to the recipient's face.
The team was able to restore and maintain blood flow to the transplanted eye by connecting it to a separate branch of arteries in the recipient’s face, rather than relying on the optic artery.
🧮 Key Results: The transplanted eye remains healthy with normal pressure and good blood flow one year after surgery.
💡 Why This May Matter: Facial disfigurement affects millions of people worldwide and this sort of pioneering procedure demonstrates the feasibility of complex, multi-organ transplants for reconstructive medicine.
🔎 Elements To Consider: While the transplanted eye remains viable, the patient cannot see through it because the research team was not able to regenerate its optic nerve. Future research must regenerate a patient’s central nervous system in order to restore the vision of transplanted eyes.
AI x Science 🤖

Credit: Google DeepMind on Unsplash
A Potential Paradigm Shift In Language Model Reasoning
OpenAI's release of its o1 series of models may be the most meaningful AI model advancement since the company released Chat GPT-4 about 2 years ago.
The reason why we are making this claim is because it appears to be the first LLM to put together all the frontier research we have faithfully shared with you throughout the year into one cohesive model, and the results are impressive.
As a reminder, the assortment of techniques that are considered best practice to maximize model reasoning include the following:
When presented with a problem to solve, have the model break it down into smaller, more manageable pieces that can be answered more reliably in isolation, similar to how a human might approach a complex task step-by-step.
Encourage the model to explore a wide range of possibilities at each step of its reasoning process, much like brainstorming multiple solutions before choosing the best path forward.
Evaluate the model's logic at each step to ensure it's maintaining a coherent line of reasoning, similar to how a teacher might check a student's work as they progress through a problem.
Implement reinforcement learning techniques to help the model learn when its rationale or answers are wrong. This allows the model to refine its problem-solving strategies over time, until it can reliably follow a path of sound reasoning that also leads to correct answers.
Introduce 'thinking time' into the model's process, where it can pause and consider multiple approaches before responding to complex and nuanced questions. This gives the model more time to search for appropriate answers and evaluate the quality of its responses.
Utilize the model itself as a judge to evaluate potential solutions. Since it's often easier to recognize a correct answer than to generate one from scratch, this approach allows the model to learn from its own successes and failures, and creates a cycle of continuous improvement.
The list goes on, but this covers the foundations of machine reasoning. While we do not know the exact points of emphasis OpenAI followed with their new o1 series to reach new reasoning breakthroughs, the first point of emphasis in their research paper mentions the importance of data for training purposes. Microsoft’s series of small Phi models are perhaps the best examples to illustrate how quality data is much more important than quantity of data, and it appears OpenAI’s partnerships with proprietary media sources are major factors in this achievement, although improvements to how they process, filter and annotate data in an automated fashion is likely even more critical to success.
The specific area of research we presume o1 is based on may have been foreshadowed by OpenAI in their Let's Verify Step By Step paper, released about two months after GPT-4. This timing suggests the paper's findings were the backbone of GPT-4's breakthrough abilities, and it represented a paradigm shift in machine reasoning at the time. In this Verify paper, OpenAI mentions "although finetuning the generator with reinforcement learning is a natural next step, it is intentionally not the focus of this work." What this means is the model of yesteryear had good evaluation systems to judge the quality of its responses, but the ‘idea generator’ portion of the system did not incorporate reinforcement learning into its operations. This gap has likely been resolved with the o1 series, and the implication means models moving forward will be more creative problem solvers and more efficient learners.
We have no idea how far this additional technique will push the field forward. From the looks of OpenAI’s performance results during training, the model has quite a bit of room to improve still before its slope of progress becomes asymptotic. There’s a lot more we have to say about this, but we need to save those thoughts for next week. Until then, let us know what you think of the new OpenAI model. How much better do you think they will get?
Our Full AI Index
AI System Predicts Brain Patterns Of Specific Behaviors: USC researchers developed an AI algorithm called DPAD that can isolate brain patterns related to specific behaviors from complex neural activity. This research is similar to a separate study we read from Howard Hughes Medical Institute about an AI system they developed to predict brain cell activities in fruit flies. These developments may eventually improve brain-computer interfaces to decode the thoughts and desires of paralyzed patients more accurately. USC. Nature.
Predicting How Proteins Adapt To Deep Sea Pressure: Researchers from Johns Hopkins University used Google's AlphaFold system to model how proteins in certain microbes respond to deep ocean pressures. The AI predicted 60% of the microbe's proteins can maintain its function at 100 megapascals, which is the approximate pressure of the ocean's deepest trenches. The team’s approach analyzed over 2,500 proteins in just a few hours, a task that would have taken decades using traditional experimental methods, and now they have a better understanding of the characteristics that allow the building blocks of life to thrive in extreme conditions. John Hopkins University. PRX Life.
Machine Learning Predicts Inflammatory Diseases In Kids: Cornell researchers developed an AI system that uses RNA in blood plasma to diagnose hard-to-differentiate pediatric inflammatory diseases. The system achieved an 80% overall accuracy at distinguishing between Kawasaki disease, Multisystem Inflammatory Syndrome in Children, viral infections, and bacterial infections, and was able to differentiate some of these diseases with up to 98% accuracy. Cornell. PNAS.
Other Observations 📰

Credit: Brett Jordan on Unsplash
Scientists Expand The Genetic Alphabet To Create New Proteins
Researchers at Scripps Research developed a way to expand the genetic alphabet and create proteins with new, synthetic building blocks. There are 20 standard amino acids found in nature, and the various combinations they can assemble into presents a vast array of proteins that do all sorts of interesting things. In spite of the massive amounts of theoretical proteins that can be found in nature, scientists still struggle to identify proteins with the precise chemical properties they need for medicine and industrial applications. One way for them to overcome this limitation is to fine-tune how a protein functions at the atomic level by adding synthetic amino acids to its existing structure.
Previous attempts to create synthetic amino acids typically involved repurposing RNA molecules. However, this approach requires extensive genome editing and may interrupt essential cellular functions in the process, so there are drawbacks to the complexity, risk, and amount of resources required to make these sorts of changes. Instead of following a similar approach, the Scripps team decided to develop a system to add a fourth amino acid to the equation. This allowed the existing genetic code to remain largely untouched and it improved the chances that a new synthetic proteins would be created.
By studying how some bacteria naturally evolved to use additional genetic instructions in certain situations, the researchers found key factors to help cells read and use the additional amino acid instructions they were introducing to the system. For example, they discovered that surrounding their new four-letter instructions with commonly-used three-letter codes helped cells incorporate the synthetic amino acid instructions more efficiently.
The team tested their method with 12 different new genetic instructions, and demonstrated they could reliably add new amino acids to specific sites in a protein without altering the rest of the cell's genome. As a proof of concept, they engineered over 100 novel cyclic peptides, each with up to three non-natural amino acids.
Just last week we mentioned how a handful of recent additions to the scientific toolkit are evolving in synergistic ways to handle proteins and edit genes with more precision than ever. It appears like another tool may be worth adding to the kit already, which is quite emblematic of today’s blistering pace of innovation. Scripps Research Institute. Nature.
Our Full Science Index
A Slate Of Clean Energy Updates: For the first time ever, zero-carbon sources made up over 40% of global electricity generation in 2023, with wind and solar contributing a record 13.9%. The momentum is clear: nearly 91% of net new global power capacity additions are coming from from wind and solar, which is up from 83% the previous year. Fossil fuels now represent just 6% of net new power capacity — the lowest level ever. Meanwhile, in the United States, domestic solar manufacturing capacity has increased by 4x in just 2 years, and new clean power capacity for utilities has risen by 91% since Q2 last year. The nation also has 73.7 GW of clean energy development projects under construction across 48 states, so new clean energy capacity is expected to compound for several more years.
Half Of Melanoma Patients Live For 10 Years With New Treatment: A 10-year follow-up study of 945 patients with advanced melanoma showed combining two immunotherapy drugs, Opdivo (nivolumab) and Yervoy (ipilimumab), sharply improved long-term survival. The median overall survival for patients receiving the combination was 71.9 months, compared to 36.9 months for Opdivo alone and 19.9 months for Yervoy alone. Remarkably, 43% of patients treated with the combination were still alive after 10 years, compared to a historical 1-year survival rate of just 25% a decade ago. Incredible. Bristol Myers Squibb. NEJM.
Media of the Week 📸
An Eel Was Swallowed Whole. Watch It Escape The Predators Stomach.
Researchers at Nagasaki University captured remarkable X-ray footage of Japanese eels escaping from the stomach of a fish. The team recorded 32 different eels that were eaten whole by a larger fish, and 9 were able to escape through the predator’s gills and esophagus after they were swallowed. Our new manta: when things get tough, be like an eel. Current Biology.
A Controller For Robots To Open & Walk Through Doors
Researchers from ETH Zurich built a controller for their robot to open and walk through various types of doors without prior knowledge of their properties. During experimental trials, their system allowed the ANYmal robot to complete this task with a 95% success rate, regardless if it was a push door, a pull door, or if the door was a different size, weight composition. This is noteworthy because it demonstrates how robots are learning to navigate human-centric environments with the help of models and hardware that are more general purpose than the technologies of yesteryear. arXiv.
A Table With 12 Walking Legs?!
Have you ever seen a 12-legged table walk before? It’s just as fascinating and creepy as you might expect. De Carpentier.
This Week In The Cosmos 🪐
September 22: The September equinox. This marks the first day of fall in the Northern Hemisphere and the first day of spring in the Southern Hemisphere.
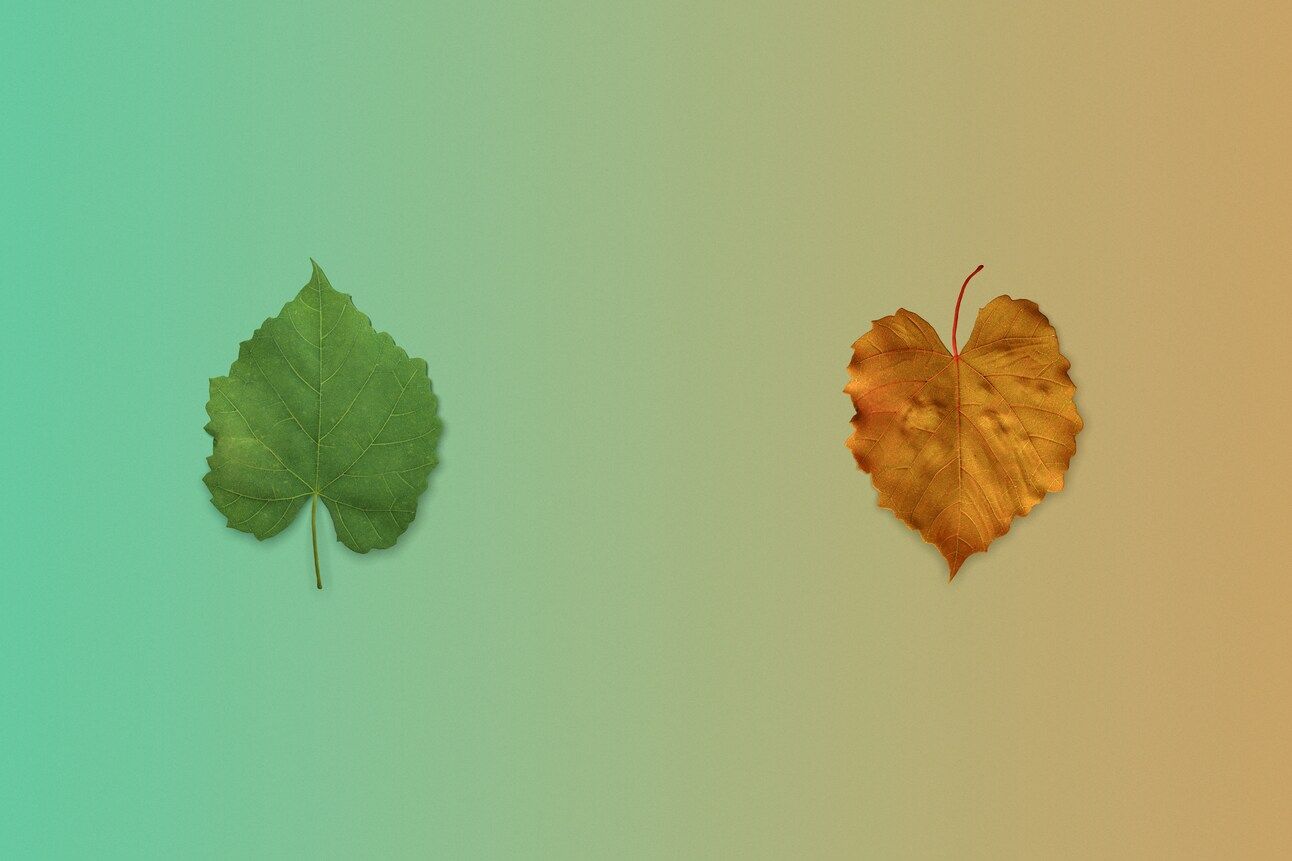
Credit: Martin Martz on Unsplash
That’s all for this week! Thanks for reading.